Artificial Intelligence & Machine Learning
,
Next-Generation Technologies & Secure Development
New Framework in o3 Models Aims to Better Align With Human Safety Values
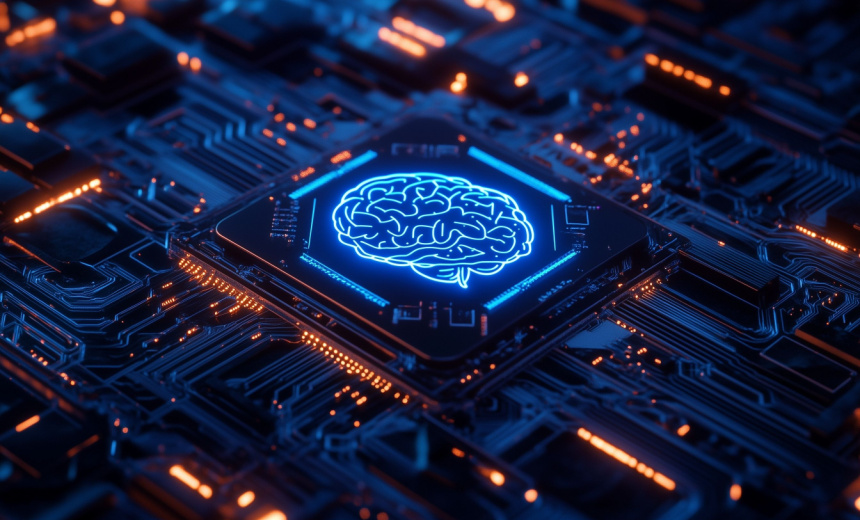
OpenAI introduced Friday its most advanced reasoning AI models while touting its safety features.
See Also: AI-Driven SOC Transformation with Cortex XSIAM
These models, o3 and o3-mini, deploy a framework called “deliberative alignment” that aims to integrate ethical reasoning in the inference phase, during which the AI model generates responses to user queries.
OpenAI says that this approach ensures a higher degree of alignment with human-defined safety values while maintaining computational efficiency.
Traditional AI training focuses on pre- and post-training interventions, such as fine-tuning models with human-labeled data or employing reinforcement learning techniques. OpenAI’s new method approaches the alignment problem by embedding safety considerations directly into the inference phase.
When a user queries the o3 model, it internally references OpenAI’s safety guidelines and breaks the question down into smaller reasoning steps through chain-of-thought reasoning. If the model is asked how to develop a nuclear bomb, it would identify the request as malicious by cross-referencing its safety policies and eventually deny the request. This internal deliberation is new, compared to other safety methodologies.
The company said it also relied on synthetic data for o3 series’ development, at a time when human-generated training data is scarce (see: AI Will Soon Exhaust the Internet. What’s Next?).
Experts have cautioned about the quality issues of synthetic data and how an overreliance on such datasets could exacerbate hallucinations. Researchers at Rice University and Stanford University have said that without fresh real data to balance out AI-generated ones, the models can “go MAD” and create a self-consuming destructive loop. They referred to this phenomenon as Model Autophagy Disorder, or MAD, and likened it to the mad cow disease that originated from feeding cattle the infected remains of other cattle.
OpenAI said it used an internal reasoning model to generate synthetic examples of chain-of-thought responses, each referencing specific elements of the company’s safety policy. Another model, referred to as the “judge,” evaluated these examples to meet quality standards.
The approach looks to address the challenges of scalability and consistency, OpenAI said. Human-labeled datasets are labor-intensive and prone to variability, but properly vetted synthetic data can theoretically offer a scalable solution with uniform quality. The method can potentially optimize training and reduce the latency and computational overhead associated with the models reading lengthy safety documents during inference.
OpenAI acknowledged that aligning AI models with human safety values remains a challenge. Users continue to develop jailbreak techniques to bypass safety restrictions, such as framing malicious requests in deceptive or emotionally charged contexts.
The o3 series models scored better than its peers Gemini 1.5 Flash, GPT-4o and Claude 3.5 Sonnet on the Pareto benchmark, which measures a model’s ability to resist common jailbreak strategies. But the results may be of little consequence, as adversarial attacks evolve alongside improvements in model defenses.
Rolling out next year, the o3 models will likely undergo further scrutiny as researchers and users assess their capabilities in real-world scenarios. OpenAI views deliberative alignment as a foundational step toward creating reasoning, ethical AI systems – essentially “the first approach to directly teach a model the text of its safety specifications and train the model to deliberate over these specifications at inference time,” OpenAI said in a blog post.
If successful, this framework could offer insight on how to better align increasingly powerful AI models with human safety values.