Artificial Intelligence & Machine Learning
,
Next-Generation Technologies & Secure Development
Researchers Detail AI’s Fabricated Facts in Healthcare, Discuss Solutions
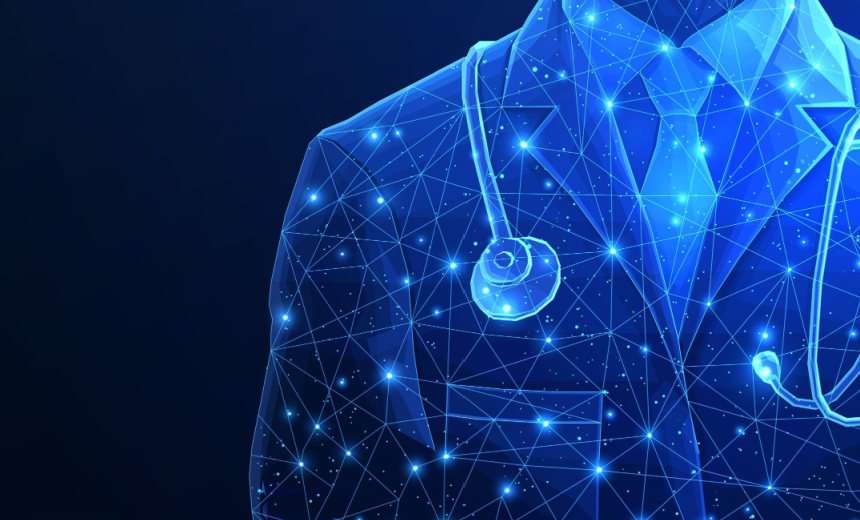
Hallucinations in artificial intelligence foundation models are pushing healthcare professionals and technologists to rethink how practitioners can safely use AI.
See Also: Capturing the cybersecurity dividend
Medical foundation models trained on vast troves of digital text and clinical data promise to revolutionize clinical decision support and medical research. But they can produce outputs that are convincingly coherent while being factually inaccurate.
Unlike generic AI hallucinations that may manifest as minor factual inaccuracies in everyday tasks, a hallucinated lab result or an erroneous diagnostic recommendation could lead to harmful interventions or missed treatments. More than two dozen experts from institutions such as MIT, Harvard Medical School and Johns Hopkins University, alongside representatives from leading tech companies, categorized medical hallucinations in a research paper and supporting GitHub repository and examined the real-world risks they pose in clinical settings.
The research team examined tasks fundamental to clinical reasoning, such as ordering patient events chronologically, interpreting lab data and generating differential diagnoses, which require precise factual recall and synthesis. Some models demonstrate a surprising aptitude for pattern recognition but often falter when precise details are crucial. Diagnostic predictions exhibited lower hallucination rates, ranging from non-existent to 22%, yet tasks demanding accurate extraction of factual details, such as chronological ordering and lab data interpretation, provoked error rates as high as nearly 25%.
The study also delineated a taxonomy of medical hallucinations that categorized errors into four types: factual errors, outdated references, spurious correlations that lead to fabricated sources or guidelines and incomplete chains of reasoning. Each category carries distinct implications for clinical practice. Factual errors directly impact a clinician’s ability to trust the AI’s recommendations, while outdated references can misguide treatment decisions based on obsolete data. Spurious correlations may lead to the endorsement of unverified medical guidelines, and incomplete reasoning can result in oversimplified or misleading conclusions. The taxonomy frames the problem and also sets the stage for developing targeted mitigation strategies.
A survey of 75 medical professionals conducted as part of the research found that 91.8% of medical practitioners had encountered hallucinations in their daily use of AI tools and 84.7% believed that such errors could adversely affect patient health. Despite these statistics, a portion of clinicians – nearly 40% of respondents – expressed a high degree of trust in AI outputs.
The survey also showed that AI tools have already become a fixture in clinical practice. Forty practitioners reported daily use of these tools, while others engaged with them several times a week or on an occasional basis. If even a fraction of the AI-generated hallucinations translates into clinical error, the stakes could be high, potentially leading to misdiagnosis, inappropriate treatment plans or even litigation against healthcare providers and technology developers alike.
The researchers caution that while the promise of enhanced diagnostic support is enticing, the models’ propensity for hallucination necessitates a cautious and measured approach. They advocated for stringent safeguards that include continual monitoring of AI outputs, enhanced training protocols that incorporate updated medical data and the inclusion of human oversight in all clinical decision-making processes.
Recent comparisons of general-purpose models showed that while some systems, such as those developed by Anthropic and OpenAI, exhibit lower rates of hallucination in diagnostic tasks, even their best outputs are not infallible.