Artificial Intelligence & Machine Learning
,
Next-Generation Technologies & Secure Development
Leaderboard Race May be More Marketing than Merit
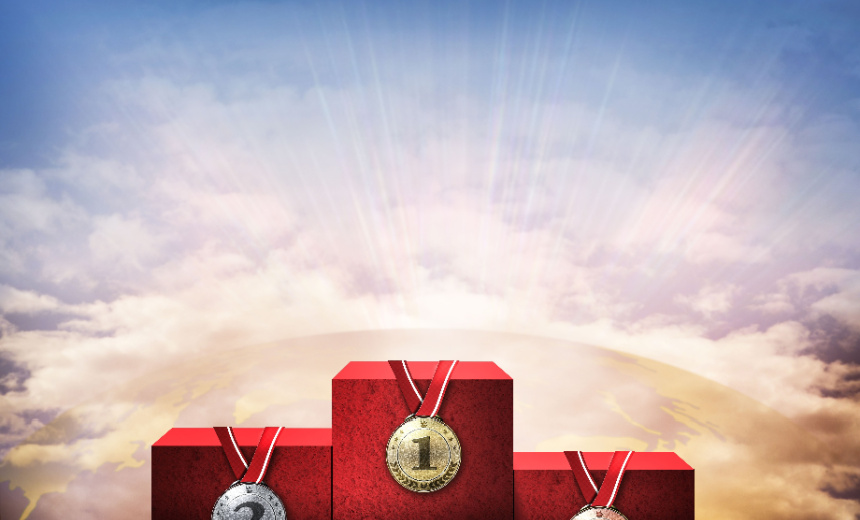
Artificial intelligence model makers routinely publish benchmark scores of their performance, but the leaderboard race may be more an exercise in marketing than an accurate reflection of the models’ abilities.
See Also: From Silos to Synergy: Gen AI Aligns IT and Security Teams
OpenAI, Google and Meta models all achieved better-than-average scores on several benchmarks the companies designed themselves. But these results may be distorted by dataset contamination, biased test construction and superficial task designs, said the European Commission’s Joint Research Center and Stanford University in separate reports that criticize current AI evaluation practices.
Stanford researchers reviewed more than 150 evaluation frameworks and found issues such as data leakage, narrow datasets and poor reproducibility. They also observed companies inflating scores through selective testing practices and inadequate dataset diversity. They voiced concern over result manipulation practices such as “sandbagging,” where models underperform on certain tests to evade scrutiny, comparing the practice to the Volkswagen emissions test scandal.
European investigators similarly identified recurring issues with benchmarks, such as unclear dataset origins. Tests fail to measure intended outcomes and overemphasize results designed to attract investors rather than provide accurate assessments, they wrote. Their study also criticized using benchmarks that cannot keep pace with rapid AI advancements and those that reinforce limited research approaches.
Understanding model failures can be more valuable than celebrating high scores, Stanford researchers said, citing previous research.
Reliable benchmarks are necessary since regulations rely heavily on them. The EU AI Act, the U.K. Online Safety Act and the US AI Diffusion Framework integrate benchmark scores into compliance standards. But JRC and Stanford researchers cautioned that current benchmarks are too inconsistent and narrow to support sound regulation.
Both reports called for AI benchmarks to meet the same standards of transparency, fairness and explainability as the models they assess.
Policymakers should encourage developers, companies, civil society groups and government organizations to articulate benchmark quality when conducting or relying on AI model evaluations and consult best practices for minimum quality assurance, the Stanford researchers said. For now, “most benchmarks are highest quality at the design stage and lowest quality at the implementation stage.”