CEO Satya Nadella Highlights DeepSeek-R1 Model, AI Cost Reductions, Future Growth
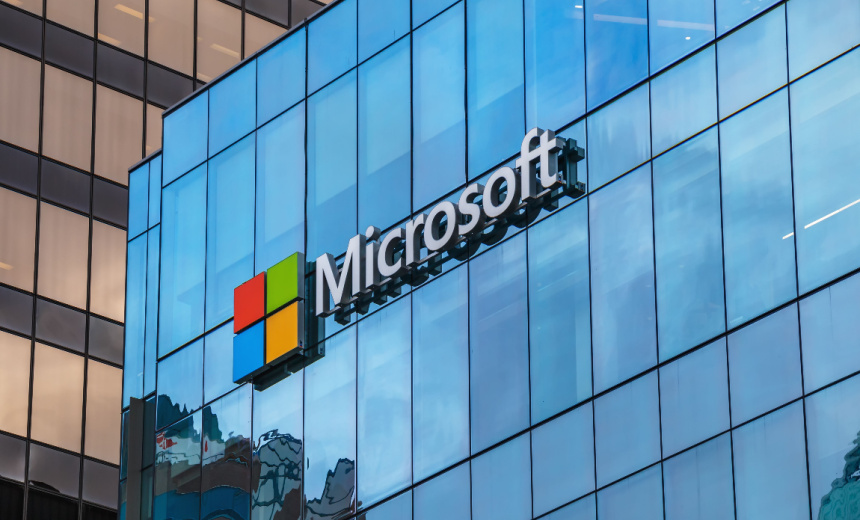
Microsoft CEO Satya Nadella said AI advancements like DeepSeek’s R1 model will drive efficiency gains, reduce inference costs and enable broader adoption of AI applications.
See Also: Uncovering Risk With Social Due Diligence
The Seattle-area software and cloud computing giant said that AI scaling laws and Moore’s Law will drive computing efficiency, cost reductions and increased accessibility, Nadella told investors Wednesday. Microsoft also made DeepSeek-R1 available on Azure AI Foundry and GitHub Wednesday to advance AI accessibility, cost-efficiency and security.
“What’s happening with AI is no different than what was happening with the regular compute cycle,” Nadella told investors. “It’s always about bending the curve and then putting more points up the curve. So there’s Moore’s Law that’s working in hyperdrive. Then on top of that, there is the AI scaling laws, both the pre-training and the inference time compute that compound and that’s all software.”
Why Improved AI Efficiency Will Drive Increased Usage
DeepSeek said it developed the technology underpinning its R1 model – which became widely available Friday – for a fraction of the money spent by U.S. developers. DeepSeek said it spent $5.6 million training its V3 model and needed just 2,048 Nvidia chips designed to be compliant with restrictions on advanced technology sales to China. This cost U.S. foundation model developers hundreds of millions of dollars (see: How China’s DeepSeek-R1 Model Will Disrupt the AI Industry).
Improvements in training and inference efficiency lead to exponential gains, Nadella said, with Microsoft observing 10x improvements per AI cycle due primarily to software optimizations rather than hardware advancements. These optimizations make AI models increasingly powerful and efficient, allowing high-end AI models that once required large-scale cloud infrastructure to now run on consumer-grade PCs.
As AI efficiency improves, Nadella said the cost of deploying and running AI models decreases, leading to higher adoption rates as businesses and developers can afford to use more AI-powered solutions. More building of AI applications will lead to increased AI adoption across industries, with small businesses and individual developers gaining access to advanced AI models without massive infrastructure investments.
“These models that are pretty powerful,” Nadella said. “It’s unimaginable to think that here we are in sort of beginning of ’25 where on the PC, you can run a model that required pretty massive cloud infrastructure. So that type of optimizations means AI will be much more ubiquitous.”
Nadella said Microsoft is continuously growing AI infrastructure globally to support the growing demand for AI applications, keeping a balance between training and inference workloads in different settings. Microsoft wants to ensure it can support both enterprise AI solutions and consumer-facing applications with cloud-based infrastructure that enables flexible AI deployments while keeping costs manageable.
“We are focused on continuously scaling our fleet globally and maintaining the right balance across training and inference as well as geo distribution,” Nadella said. “From now on, it’s a more continuous cycle governed by both revenue growth and capability growth, thanks to the compounding effects of software-driven AI scaling laws and Moore’s Law.”
How DeepSeek on Azure Fits Into Microsoft’s AI Strategy
Nadella said that optimizing inference costs is essential for AI adoption since AI models won’t generate significant demand if running them is too expensive. Microsoft ensures optimal fleet management by keeping AI infrastructure flexible so it can continuously integrate the latest hardware and software advancements. Instead of buying massive AI hardware stacks at once, Microsoft continuously upgrades.
“We are working super hard on all the software optimizations, not just the ones that come because of what DeepSeek has done, but all the work we have done to, for example, reduce the prices of GPT models over the years in partnership with OpenAI,” Nadella said. “You’ve got to have that optimization so that inferencing costs are coming down and they can be consumed broadly.”
Microsoft said making DeepSeek-R1 available on Azure AI Foundry and GitHub is part of the company’s larger strategy to provide accessible AI models to developers since pre-trained AI models are becoming easier to integrate into business applications with minimal infrastructure requirements. The cloud-based model catalog allows enterprises to quickly deploy AI while maintaining security and compliance.
“This rapid accessibility—once unimaginable just months ago—is central to our vision for Azure AI Foundry: bringing the best AI models together in one place to accelerate innovation and unlock new possibilities for enterprises worldwide,” Asha Sharma, Microsoft’s corporate vice president of AI Platform, wrote in a blog post Wednesday.
Microsoft has built in several security measure to DeepSeek-R1 including automated red teaming, content filtering and model behavior assessments to ensure that AI models deployed on Microsoft’s platform adhere to ethical standards and mitigate risks, Sharma wrote. These safeguards reduce risks for businesses, Sharma wrote, ensuring that AI can be deployed confidently and responsibly.
“We are committed to enabling customers to build production-ready AI applications quickly while maintaining the highest levels of safety and security,” Sharma wrote in the blog post. “DeepSeek-R1 has undergone rigorous red teaming and safety evaluations, including automated assessments of model behavior and extensive security reviews to mitigate potential risks.”