DeepSeek’s Reasoning AI and Inference Scaling Driving Massive Demand for Compute
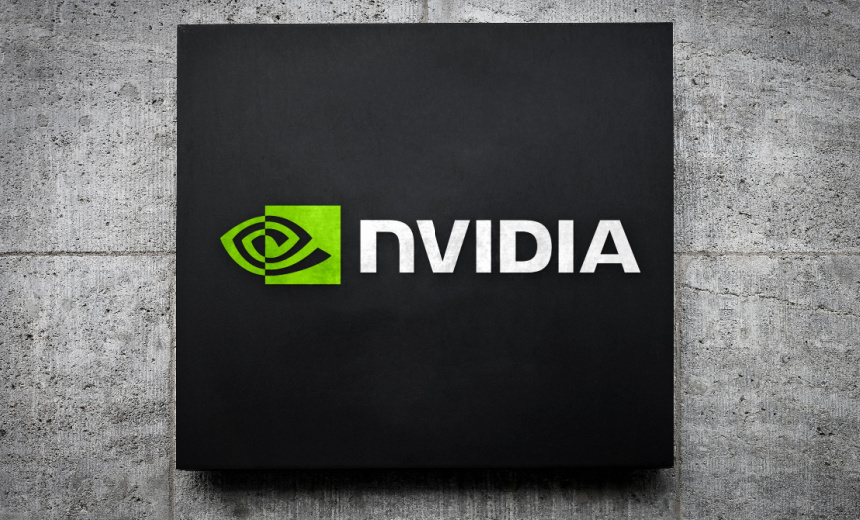
Nvidia founder and CEO Jensen Huang said the open-source release of DeepSeek-R1 has accelerated demand for compute by driving widespread adoption of reasoning AI techniques.
See Also: The Comprehensive Guide for a Viable BYOD Policy
The Silicon Valley-based chip giant said the significant computational requirements for post-training customization and inference scaling now surpass pre-training compute demands, Huang told investors. Reasoning AI uses significantly more computational power than traditional one-shot inference models, while reinforcement learning, fine-tuning and model distillation use more compute than pre-training.
“AI is evolving beyond perception and generative AI into reasoning,” Huang said Wednesday. “With reasoning AI, we’re observing another scaling law—test time scaling, more computation. The more the model thinks, the smarter the answer.”
Why Reasoning Models Need More Compute than Traditional AI
Nvidia’s sales in the quarter ended Jan. 26, 2025, surged to $39.33 billion, up 77.9% from $22.1 billion the year prior. The company’s net income jumped to $22.09 billion, or $0.89 per share, up 79.8% from $12.29 billion, or $0.49 per share, the year prior. Nvidia’s stock fell $1.96 – or 1.49% – to $129.32 per share in after-hours trading. The company’s stock is down 9.3% since the release of DeepSeek-R1 (see: Singapore to Probe DeepSeek’s High-End Nvidia Chip Purchases).
Models like OpenAI’s o3, DeepSeek-R1 and Grok-3 demonstrate how AI is shifting from perception and generative models to long-thinking reasoning models that require significantly more compute power, Huang said. These models can solve complex problems, make strategic decisions, and apply logical reasoning, but require 100 times more compute per task than traditional inference-based AI, he said.
“The scale of post-training and model customization is massive and can collectively demand orders of magnitude more compute than pre-training,” Huang said. “Our inference demand is accelerating, driven by test time scaling and new reasoning models.”
Data centers historically have been built around CPU-based architectures and designed for traditional software applications, but must now prioritize GPU-accelerated computing given the rise of machine learning and AI-based software. The data centers of the future will be AI factories, meaning they’ll be primarily optimized for training and deploying AI models rather than simply storing and processing data.
“Going forward, data centers will dedicate most of CapEx to accelerated computing and AI,” Huang said. “Data centers will increasingly become AI factories, with every company either renting or self-operating. We have a fairly good line of sight of the amount of capital investment that data centers are building out towards. Going forward, the vast majority of software is going to be based on machine learning.”
What AI Applications Are Coming Down the Pike
Huang said Nvidia’s Blackwell architecture is designed to seamlessly transition between pre-training, post-training and inference scaling to ensure that AI workloads can be processed more effectively. A high-speed interconnect facilitates large-scale AI processing by connecting GPUs in an optimized manner, helping Blackwell process reasoning AI models 25 times faster than Nvidia’s old architecture.
“We defined Blackwell for this moment – a single platform that can easily transition from pre-trading, post-training and test-time scaling,” Huang said.
While consumer AI and search-based generative AI have seen rapid adoption, Huang said the next wave of AI applications are only just beginning to take off. He said the new era will be defined by AI-powered autonomous agents capable of decision-making, planning, and executing complex tasks without human intervention. Governments and companies will develop nation-specific AI ecosystems, ensuring privacy.
“The next wave is coming – Agentic AI for enterprise, physical AI for robotics, and sovereign AI as different regions build out their AI for their own ecosystems,” Huang said. “Each one of these is barely off the ground, but we can see them because we are at the center of much of this development, and we see great activity happening in all these different places.”