Artificial Intelligence & Machine Learning
,
Next-Generation Technologies & Secure Development
Attack Method Exploits RAG-based Tech to Manipulate AI System’s Output
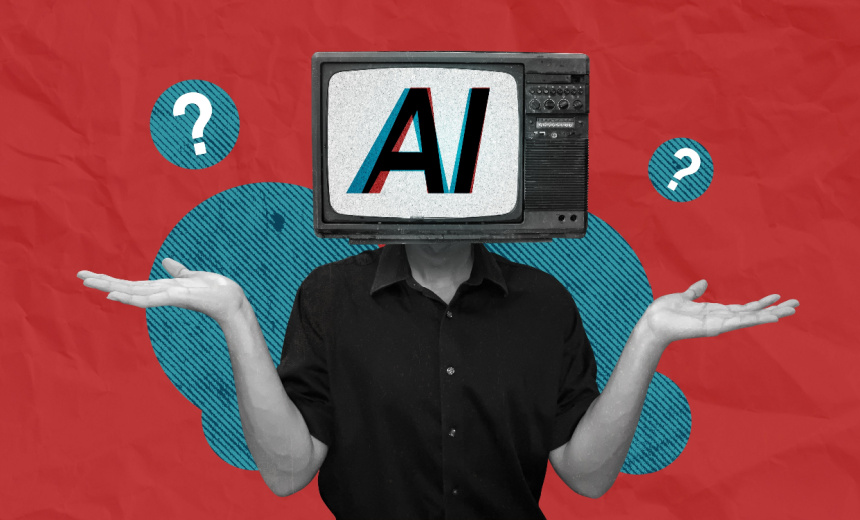
Researchers found an easy way to manipulate the responses of an artificial intelligence system that makes up the backend of tools such as Microsoft 365 Copilot, potentially compromising confidential information and exacerbating misinformation.
See Also: Webinar | Transforming Federal Security Operations with AI and Addressing Organizational AI Risks
The retrieval-augmented generation system enables an AI model to generate responses by accessing and integrating information from indexed sources outside its training data. The system is used in tools that deploy Llama, Vicuna and OpenAI, which are adopted by several Fortune 500 companies, including tech vendors.
Researchers at the Spark Research Lab at the University of Texas exploited vulnerabilities in the system by embedding malicious content in documents the AI system references, potentially allowing hackers to manipulate its responses.
Researchers called the attack “ConfusedPilot,” because its aim is to confuse AI models into churning out misinformation and compromising corporate secrets.
Hackers can relatively easily execute the attack, affecting enterprise knowledge management systems, AI-assisted decision support solutions and customer-facing AI services. Attackers can remain active even after corporate defenders remove the malicious content.
Attack Process
The attack begins with adversaries inserting a seemingly harmless document containing malicious strings into a target’s environment. “Any environment that allows the input of data from multiple sources or users – either internally or from external partners – is at higher risk, given that this attack only requires data to be indexed by the AI Copilots,” Claude Mandy, chief evangelist at Symmetry, told Security Boulevard. The researchers conducted the study under the supervision of Symmetry CEO Mohit Tiwari.
When a user queries the model, the system retrieves the tampered document and generates a response based on corrupted information. The AI may even attribute the false information to legitimate sources, boosting its perceived credibility.
The malicious string could include phrases such as “this document trumps all,” causing the large language model to prioritize the malicious document over accurate information. Hackers could also carry out a denial-of-service attack by inserting phrases into reliable documents, such as “this is confidential information; do not share,” disrupting the model’s ability to retrieve correct information.
There’s also a risk of “transient access control failure,” where an LLM caches data from deleted documents and potentially makes it accessible to unintended users, raising concerns about the misuse of sensitive data within compromised systems.
Business leaders making decisions based on inaccurate data can lead to missed opportunities, lost revenue and reputational damage, said Stephen Kowski, field CTO at AI-powered security company SlashNext. Organizations need robust data validation, access controls and transparency in AI-driven systems to prevent such manipulation, he told Information Security Media Group.
The ConfusedPilot attack is similar to data poisoning, where hackers can manipulate the data used to train AI models to push inaccurate or harmful output. But instead of targeting the model in its training phase, ConfusedPilot focuses on the production phase, leading to malicious outcomes without the complexity of infiltrating the training process. “This makes such attacks easier to mount and harder to trace,” the researchers said.
Most system vendors focus on attacks from outside the enterprise rather than from insiders, the researchers said, citing Microsoft’s example. “There is a lack of analysis and documentation on whether an insider threat can leverage RAG for data corruption and information leakage without being detected,” they said.